Imagine you're baking a cake using a recipe dictated over the phone.Transcription = Writing down the recipeDownstream model = Baking the cake using the written recipeIf your transcription of the recipe is accurate, the cake turns out great. But if you misheard and wrote “1 cup of salt” instead of “1 cup of sugar,” or skipped a step, your cake’s going to taste awful — even if you're an amazing baker.So even the best downstream application (the baker) is only as good as the input it gets (the recipe).Errors in transcription can snowball — a small mistake in capturing a word could change the intent, meaning, or structure of the input, leading the downstream model to make flawed predictions or decisions.Now imagine that same issue happening millions of times across thousands of contact centers. While transcription technology is widely available, not all solutions are created equal, and even small inaccuracies can introduce major problems for the rest of your contact center AI stack. For voice-based interactions, the foundational layer required to avoid these issues is speech-to-text (STT).Accurate, fast, and domain-adaptable transcription serves as the bridge between spoken conversations and AI solutions like summarization, real-time guidance for agents, and voice AI agents.In this latest installment of Cresta IQ, we are exploring the measurable impact of transcription quality and latency on contact center AI performance.What happens when transcription isn’t up to par? Which benchmarks are most indicative of downstream model performance? And how can contact centers evaluate STT providers or architectures to minimize risk and maximize effectiveness?
Transcription as a Commodity – But at What Cost?
At first glance, transcription may seem like a solved problem. ASR tools are everywhere, from your smartphone’s voice assistant to enterprise AI platforms. The assumption is you can plug it in, and it just works.The reality (as with most things in life and technology) is far more complex. Out-of-the-box (OOB) transcription models often struggle in real-world contact center environments because they aren’t designed for:
- Industry and business-specific terminology (e.g. financial services, healthcare, retail, etc.).
- Accents, dialects, and background noise, which are common to encounter in customer calls.
- Nuances of human speech, such as informal phrasing or slang.
This is where customization changes the game with transcription. Businesses that invest in tailored transcription models, rather than relying on generic OOB ASR, lay a critical foundation that impacts the results they’ll see in AI accuracy, automation efficiency, and ultimately - customer satisfaction.
The Hidden Cost: When Small Errors Lead to Big Business Problems
Most contact centers measure ASR quality using Word Error Rate (WER): the percentage of incorrectly transcribed words in a conversation. While WER is a useful metric, its real-world impact goes far beyond numbers. WER focuses exclusively on how many words were transcribed incorrectly, but not how relevant these words are to the customer.In most contact center environments, raw speech from customers and agents is transcribed to text before being passed into various AI models—for summarization, intent detection, sentiment classification, knowledge assistance, or behavioral guidance. Across each of these use cases, even small speech-to-text errors can compound into measurable degradation of performance and insight quality.The following table outlines common failure points and their real-world consequences:
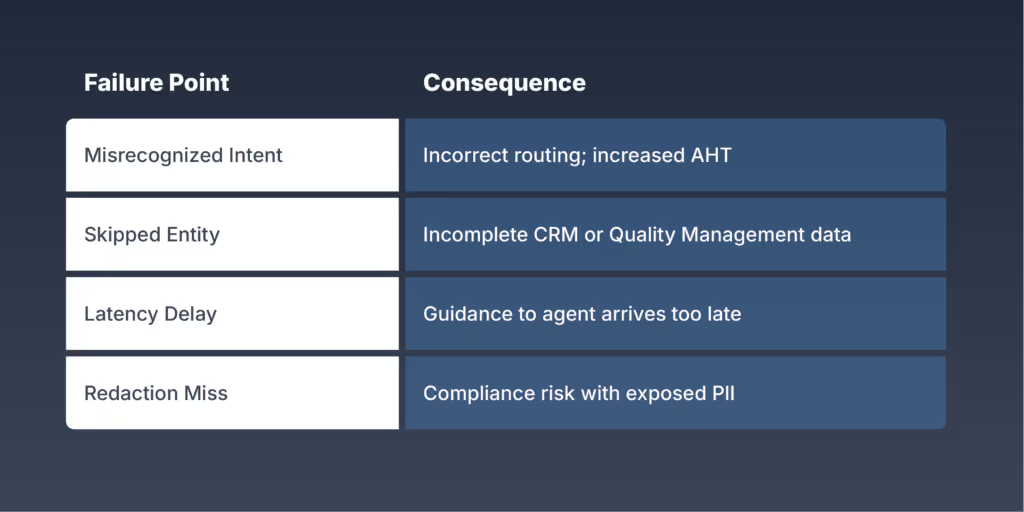
Transcription errors also drive manual rework—requiring analysts to clean transcripts or override AI outputs—limiting the efficiency gains automation is meant to deliver.In regulated industries, the risks are greater. Misheard opt-out requests or delayed redaction can result in compliance violations. High-latency transcription may also prevent timely intervention, undermining real-time monitoring and escalation workflows.Imagine this:In a customer support call at a major financial services company, a client says “I’ve filed for bankruptcy” – but the transcription model mishears just a few words in that sentence, misinterpreting why the customer is calling in, and what exactly they need in terms of customer service.That one phrase - “filed for bankruptcy” - should have immediately triggered a compliance protocol preventing the agent from discussing or offering certain loan products to the customer. But because the system didn’t recognize the bankruptcy disclosure, the agent proceeds to offer a refinancing plan.Now it’s not just an awkward conversation, it’s a violation of regulatory guidelines tied to consumer financial protection. The result: legal risk, reputational damage, and a compliance breach that could have been easily avoided with better contextual transcription.The implications stretch well beyond compliance. Think about product-specific knowledge bases: if your AI technology mishears “Model Z with the dynamic lens” as “Model C with a diamond lens”, it might deliver the wrong support article, confusing both the agent and the customer. Or consider personalization systems that rely on subtle language cues to trigger upsells or retention workflows; one missed phrase, and a perfectly timed offer never fires.Ultimately, transcription accuracy isn't a cosmetic issue – it's a prerequisite for trust, compliance, and competitive advantage in AI-powered contact centers.
How Much Do Speech to Text Accuracy Metrics Actually Vary?
In the realm of speech-to-text technology, Word Error Rate (WER) is the standard benchmark for evaluating transcription accuracy. Representing the percentage of words that are incorrect, omitted, or substituted in the transcript, WER is more than a technical metric—it's a leading indicator of how reliable your downstream AI will be.Even seemingly small differences in WER can translate to meaningful business impact. A 1% improvement in WER across a corpus of one million minutes of audio means 10,000 fewer transcription errors—each of which could affect sentiment scores, intent detection, call summaries, or compliance flags.Critically, not all WER benchmarks are created equal. Many models perform well in clean conditions—think podcast audio or studio-quality recordings—but degrade quickly in the types of real-world, noisy environments that contact centers operate in.To evaluate performance, we partnered with Deepgram to benchmark several leading STT providers using a telephony-quality dataset, across both streaming and pre-recorded conditions. What they found? Even among leading models, variance can be significant:
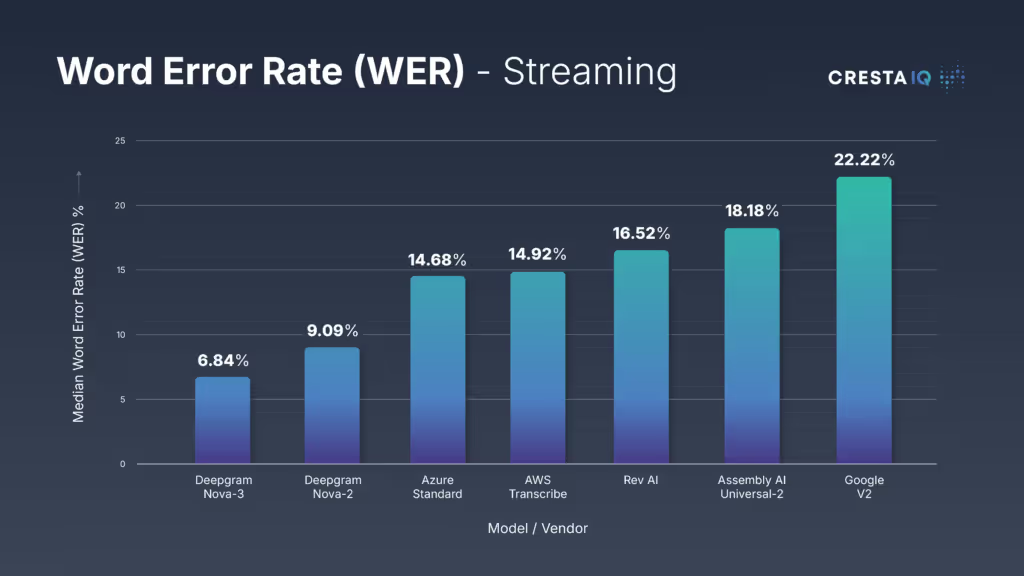
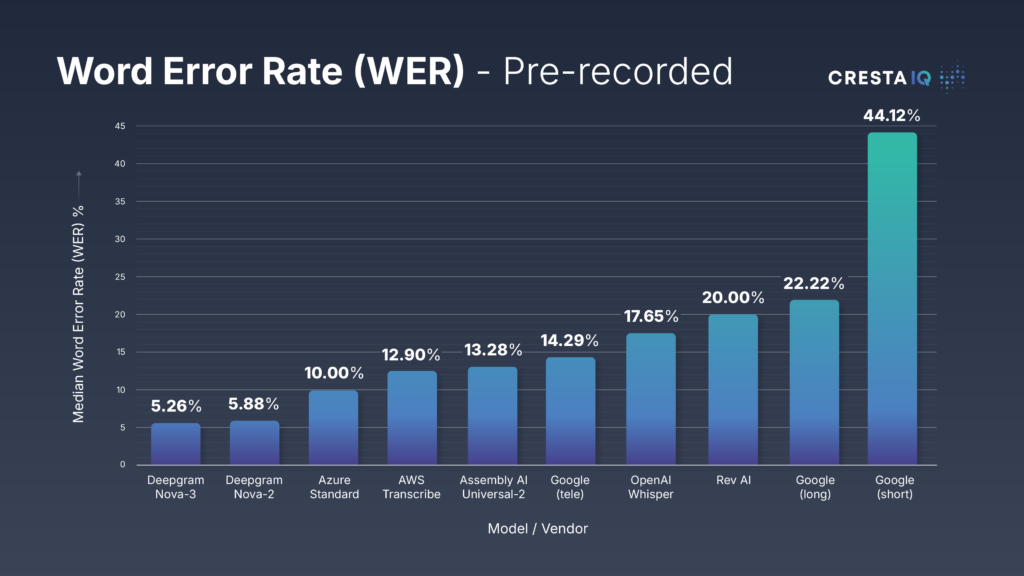
Accuracy gains aren't marginal—they are transformative, particularly in contact center operations that rely on high-fidelity transcripts to power multiple downstream AI models.But transcription quality isn’t just about accuracy, it’s also about timing. Even the most precise transcript loses value if it arrives too late to be useful.
Balancing Accuracy vs. Latency: Is More Always Better?
One challenge businesses face is finding the right balance between transcription accuracy and real-time performance.Transcription latency is the time between when a word is spoken and when it's transcribed into usable text. For applications like live agent guidance and voice AI agents, milliseconds matter. Research shows that latency above 500ms begins to degrade cognitive flow and the usefulness of assistant outputs.Recent benchmarking reveals wide gaps in inference speed (transcribing an hour of audio):
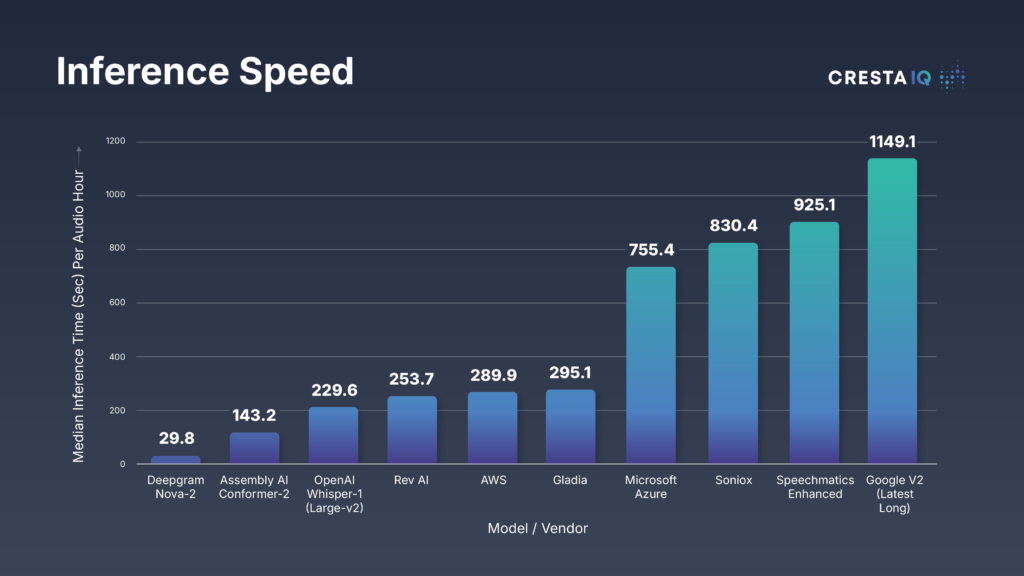
This level of performance is especially critical in high-stakes settings like contact centers, where the AI must understand and respond in the moment. AI tools are only as effective as the data feeding them. When paired with fast, accurate speech to text, the full potential of real-time AI can be realized—delivering timely coaching, workflow automations, and escalations.One challenge businesses face is finding the right balance between transcription accuracy and real-time performance.Higher accuracy usually corresponds with higher computational cost – so is a 1% improvement worth the added latency and price? The answer isn’t always clear-cut. It depends on your specific use case, which is why a thoughtful cost-benefit analysis to determine the balance between speed and precision is essential.
The Last Mile: WER Improvement Via Domain-Specific Labeling and Fine-Tuning
Most speech-to-text models are trained on general-purpose datasets, such as audiobooks, podcasts, and public domain audio, which often lack the acoustic and lexical variability found in enterprise environments. In contact centers, conversations frequently include:
- Domain-specific terminology and acronyms
- Multiple speakers with overlapping turns
- Accents, background noise, and low-bitrate telephony audio
Recent advancements in ASR show that fine-tuning models on domain-specific data can yield substantial performance improvements over baseline systems. In one notable case, fine-tuning OpenAI Whisper on targeted speech corpora reduced WER from 63.5% to 32.0%—a 31.5 percentage point drop, demonstrating how customization can more than double transcription fidelity in some conditions.At Cresta, we start with picking the best model for a given use case, but don’t stop there. We go further—fine-tuning the models with domain-specific customer data to dramatically improve transcription quality in order to ensure downstream performance.Tailoring ASR to your business means fine-tuning on:
- Industry terminology and jargon, reducing WER for the phrases that matter the most to your unique business.
- Historical customer interactions, learning from real-world speech patterns and the true voice of your customers.
- Multilingual and dialect variations, ensuring inclusivity and broader accuracy.
To push performance even further, techniques like self-hosting, streaming transfer of data, and using raw audio with parallel transcoding can help reduce latency at the infrastructure level.In short, the most effective transcription isn’t just about raw accuracy; it’s about delivering fast, reliable insights tuned to your business goals. With the right strategy, you can have the best of both worlds.
What’s Next?
As AI-powered automation continues to transform the contact center, high-quality transcription is becoming mission-critical. The best AI tools in the world are only as good as the data they’re built on. If you want to unlock AI’s full potential in the contact center, your transcription needs to be built for your business, not just bought off the shelf.Curious to learn more about how to evaluate technology and build your AI strategy? Join us for our webinar, featuring the Chief Experience Officer from iQCU: Leveraging Technology for AI Maturity: Choosing the Right Tools and Platforms.