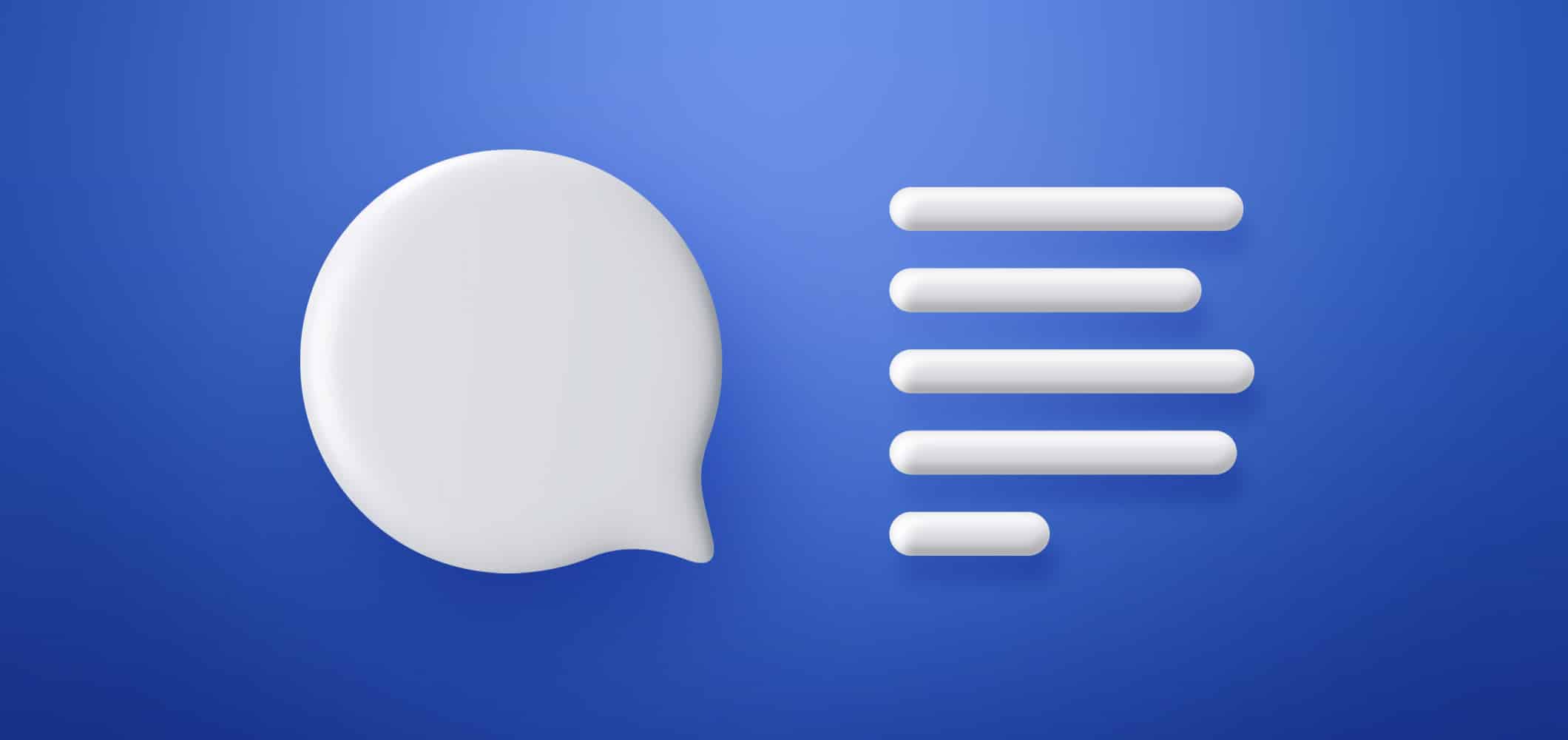
Guest post by Hasan Jilani, Director of Product Marketing at Deepgram
The Role of Speech to Text in the AI-Powered Contact Center
Contact centers are rapidly evolving as artificial intelligence (AI) becomes embedded across the customer experience stack. AI agents now assist with routine inquiries, reduce wait times, guide human agents during live calls, and generate actionable insights through post-call analytics.
As these systems grow more capable, their effectiveness hinges on one key factor: the quality of the data they consume. For voice-based interactions, that foundational layer is speech-to-text.
Accurate, fast, and domain-adaptable transcription serves as the bridge between spoken conversations and AI understanding. Without it, downstream systems like summarization, sentiment analysis, and agent assist risk being misinformed or delayed.
This blog explores the measurable impact of transcription quality on contact center AI performance. What happens when speech-to-text accuracy or latency falls short? Which benchmarks are most indicative of downstream model performance? And how can contact centers evaluate STT providers or architectures to minimize risk and maximize effectiveness?
Drawing on benchmarking data and real-world use cases, this analysis helps practitioners evaluate speech-to-text systems with the same rigor applied to any critical layer of AI infrastructure.
What’s at Stake: How Speech to Text Quality Impacts Downstream AI
In most contact center environments, raw speech from customers and agents is transcribed to text before being passed into various AI models, such as summarization, intent detection, sentiment classification, escalation handling, or compliance auditing. At each of these steps, even small speech-to-text errors can compound into measurable degradation of performance and insight quality.
Key failure modes include:
- Sentiment skew: Sentiment analysis models rely on the transcription of emotionally weighted language, such as words that signal frustration, delight, confusion, or urgency. Misrecognizing phrases like “this is ridiculous” as “this is red licorice” not only inverts the intended meaning but also distorts sentiment scores across QA dashboards and analytics reports. When this happens at scale, it leads to a systemic misunderstanding of customer satisfaction trends.
- Intent failure: Intent recognition systems depend on domain-specific keywords, including terms like “cancel,” “upgrade,” “billing,” or “representative,” to classify customer needs. If these are misheard or skipped in the transcription layer, the AI can misroute the interaction, fail to automate a task, or provide an irrelevant recommendation to an agent. The result is longer handle times, unnecessary escalations, and a frustrating experience for the customer.
- Topic dilution: Topic modeling algorithms use word frequency and co-occurrence patterns to identify dominant conversation themes. If key nouns or concepts are transcribed incorrectly or missed entirely, the model may misidentify or fail to surface critical topics. This undermines efforts to track common pain points, identify coaching opportunities, or discover emerging customer trends.
These risks aren’t just theoretical. In production environments, they manifest as reduced trust in AI-generated insights, missed opportunities for intervention, and weaker alignment between customer expectations and organizational responses.
How Much Do Speech to Text Accuracy Metrics Actually Vary?
In the realm of speech-to-text technology, Word Error Rate (WER) is the standard benchmark for evaluating transcription accuracy. Representing the percentage of words that are incorrect, omitted, or substituted in the transcript, WER is more than a technical metric. It is a leading indicator of how reliable your downstream AI will be.
Even seemingly small differences in WER can translate to meaningful business impact. A 1% improvement in WER across a corpus of one million minutes of audio means 10,000 fewer transcription errors, each of which could affect sentiment scores, intent detection, call summaries, or compliance flags.
Critically, not all WER benchmarks are created equal. Many models perform well in clean conditions, such as podcast audio or studio-quality recordings, but degrade quickly in the types of real-world, noisy environments that contact centers operate in.
To evaluate performance more realistically, WER benchmarking was based on a dataset comprising 2,703 audio files across nine distinct domains, totaling 81.69 hours of recorded speech. The dataset reflects telephony-quality audio and includes both streaming and pre-recorded conditions:
These benchmarks highlight several key takeaways:
- Deepgram Nova-3 consistently outperforms all competitors, including hyperscalers like Google, Azure, and AWS, in both streaming and batch scenarios.
- Nova-3 delivers 54% lower WER than AWS in streaming conditions and up to 2x the accuracy of OpenAI Whisper in pre-recorded use.
- Accuracy gains aren’t marginal; they are transformative, particularly in contact center pipelines that rely on high-fidelity transcripts to power multiple downstream AI models.
In a noisy, high-volume environment, choosing a lower WER model is not just a technical preference; it is a strategic lever for improving every AI-driven outcome from agent assist to sentiment dashboards.
Why Speech to Text Latency Can Break Real-Time AI
Latency plays a critical role in determining how fast and intelligent real-time AI feels in the contact center. Whether you’re powering agent assist, voicebots, or live QA dashboards, transcription delay directly affects the relevance and perceived intelligence of the system.
Transcription latency is the time between when a word is spoken and when it’s transcribed into usable text. For applications like live agent guidance and real-time analytics, milliseconds matter. Research shows that latency above 500ms begins to degrade cognitive flow and the usefulness of assistant outputs.
When transcription is delayed:
- Agent assist tools lag behind the conversation, making suggestions feel stale or off-topic
- Voicebots lose their responsiveness and natural cadence
- Live dashboards show incomplete or outdated data, undermining real-time decision-making
Recent benchmarking reveals wide gaps in inference speed (transcribing an hour of audio):
Nova-2’s processing speed is 5–40x faster than many alternatives. Deepgram’s Nova-3 offers similar performance to Nova-2, and both models deliver streaming speech-to-text with average end-to-end latency under 300 milliseconds, well below the 500 ms usability threshold. This positions them among the fastest STT models available for latency-sensitive contact center applications.
This level of performance is especially critical in high-stakes settings like contact centers, where the AI must understand and respond in the moment. Tools like Cresta’s Agent Assist are only as effective as the data feeding them. When paired with fast, accurate speech-to-text, the full potential of real-time AI can be realized, delivering timely coaching, escalation insights, and live QA without interruption.
Customizing Speech to Text for Contact Center Use Cases
Most speech-to-text models are trained on general-purpose datasets, such as audiobooks, podcasts, and public domain audio, which often lack the acoustic and lexical variability found in enterprise environments. In contact centers, conversations frequently include:
- Domain-specific terminology and acronyms
- Multiple speakers with overlapping turns
- Accents, background noise, and low-bitrate telephony audio
These factors contribute to elevated Word Error Rates (WER) in generic ASR systems, which in turn degrade the performance of downstream tasks like intent recognition, agent assist, and sentiment analysis.
Recent advancements in ASR show that fine-tuning models on domain-specific data can yield substantial performance improvements over baseline systems. In one notable case, fine-tuning OpenAI Whisper on targeted speech corpora reduced WER from 63.5% to 32.0%–a 31.5 percentage point drop, demonstrating how customization can more than double transcription fidelity in some conditions.
Deepgram supports a multi-tiered approach to customization, offering increasingly granular control over model behavior:
Keyterm Prompting
- Boost domain-specific vocabulary (e.g., product names, acronyms)
- No retraining needed
- Available in Deepgram’s Nova-3 model, this feature allows for fast adaptation to brand-specific language or regional terminology without any downtime.
A benchmark comparing Nova-2 (baseline) to Nova-3 with prompting (fine-tuned) illustrates the impact: with prompting enabled, keyterm recognition jumped from 44% to 90%, more than doubling accuracy for critical vocabulary.
Model Adaptation Loops
- Automatically retrain using curated or synthetic audio
- Improves domain fit over time
- Deepgram’s Enterprise Runtime uses a three-factor adaptation system: curating high-value training data, generating synthetic examples to improve edge case coverage, and automatically retraining models to keep pace with evolving customer conversations.
Custom Models
- Tailored to your data using supervised training
- Beneficial for organizations with high call volumes in niche or regulated industries
- Deepgram offers custom model development services for teams requiring tighter control over vocabulary and performance in specialized environments
Fine-tuning and domain adaptation have proven effective in real-world deployments. One example: a veterinary voice platform achieved a 625% improvement in key term recognition by applying a prompting-based enhancement, significantly improving transcript quality for domain-specific vocabulary.
Similarly, Revenue.io integrated Deepgram’s customized speech-to-text into its contact intelligence stack to improve transcript accuracy, leading to better CRM sync, more effective sales coaching, and stronger alignment between voice data and automation.
These capabilities help reduce long-tail WER, improve downstream model fidelity, and deliver more accurate, actionable insights without extensive engineering lift.
The Hidden Costs of Suboptimal Transcription Accuracy
The consequences of low transcription accuracy extend well beyond word-level mismatches, and they ripple through the entire AI stack of modern contact centers. Models that depend on accurate transcripts for intent detection, compliance monitoring, and real-time guidance are especially sensitive to input quality. When STT errors occur, the downstream outputs of these models become misaligned with the actual conversation.
The following table outlines common failure points and their real-world consequences:
Transcription errors also lead to manual rework, as analysts must clean transcripts or override AI outputs, ultimately limiting the efficiency gains that automation is intended to deliver.
For example, CallTrackingMetrics reported that 40% of their transcripts were unusable before switching to Deepgram. After the change, usable transcripts jumped to over 90%, unlocking analytics and QA capabilities that were previously unreliable.
Similarly, a healthcare contact center using Five9 doubled its IVR authentication success rate after adopting a lower-WER STT system. Previously, the ASR routinely misrecognized spoken account numbers, forcing calls to live agents. Improved transcription enabled more customers to complete self-service authentication, reducing escalations and improving containment.
In regulated industries, the risks are greater. Misheard opt-out requests or delayed redaction can result in compliance violations. High-latency transcription may also prevent timely intervention, which undermines real-time monitoring and escalation workflows.
Ultimately, transcription accuracy isn’t a cosmetic issue; it is a prerequisite for trust, compliance, and competitive advantage in AI-powered contact centers.
Speech-to-Text as the Interface to AI
Speech-to-text is not just a technical component; it is the foundational interface between the spoken customer experience and AI-driven understanding. Its accuracy and latency directly impact every layer of the contact center intelligence stack, from real-time agent assist and compliance detection to post-call analytics and summarization.
The data presented in this blog reinforces that transcription quality is a strategic variable. When speech-to-text systems fall short, whether due to high word error rates or excessive latency, those shortcomings propagate downstream, leading to flawed insights, missed automation opportunities, and lower customer satisfaction. Conversely, accurate and timely transcription enables more responsive, trustworthy AI.
One example of this layered architecture in practice is the partnership between Deepgram and Cresta. Deepgram provides the transcription infrastructure, optimized for accuracy, speed, and adaptability, while Cresta applies real-time intelligence to deliver in-the-moment coaching, escalation handling, and insight generation. Together, they illustrate how modular, best-in-class systems can be combined to unlock measurable improvements in contact center performance.
Ultimately, speech-to-text is not an afterthought. It is core infrastructure for voice AI. As enterprises scale their use of automation in the contact center, they should evaluate transcription not just as a commodity service but as a critical enabler of accuracy, speed, and AI effectiveness.